
This summer school was the second edition of MOOD’s capacity building event, planned for improving knowledge on epidemic intelligence for disease surveillance, including identification of re-emerging infectious diseases and antimicrobial resistance, the changing drivers fueling disease spread, and surveillance and early warning techniques in pandemic threats. During these 6 days, at the MEDILS institute in Split, Croatia, hands-on skills improvement through the use of MOOD tools were highlighted, as well as online materials such as data processing tutorials.
All the lectures were recorded and are hosted on the Leibniz Information Centre for Science and Technology and University Library (TIB AV-Portal), which provides ad-free videos on science, research, industry and business with literature and information.
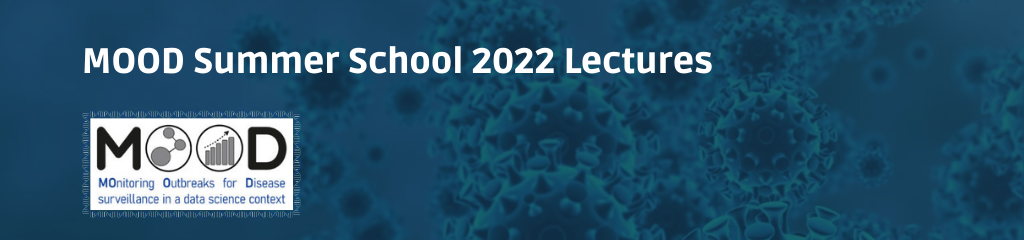
All the lectures are hosted on the Leibniz Information Centre for Science and Technology and University Library (TIB AV-Portal), which provides ad-free videos on science, research, industry and business with literature and information.
In this video tutorial, Timothee Dub and Henna Mäkelä (Finnish Institute for Health and Welfare, Finland ) discussed the basics of infectious disease surveillance (event-based and indicator-based surveillance, active versus passive surveillance), as well as the advantages and limitations of each type of systems, followed by the example of how surveillance activities for TBE are conducted in Finland. By the end of this lecture, participants should be aware of the limitations and quality issues that can occur when using surveillance data for comparison and/or modelling.
In this session, Facundo Muñoz (Cirad, France) describex tools and workflows to cumulatively improve the reproducibility of analyses performed in R. R is a mature, world-class, open-source statistical computing and data-analysis platform with a huge community of users from all areas of science and industry. Yet, most researchers rely only on its most basic scripting features, missing the opportunity to unleash its full potential, in particular concerning reproducible-research workflows. Specifically, we discuss encoding and platform-specific packages, the advantages of organising code into functions, using project-directories and relative paths, reproducible reports with RMarkdown, controlling package versions with Renv, organising code into a pipeline with targets, keeping track of changes from various collaborators with git, reproducibly publishing results with Continuous Integration in Git(Hu|La)b pages, reproducing the complete environment with docker, and controlling versions of the complete software stack with GNU Guix.
This lecture, Francesca Dagostin (Fondazione Edmund Mach, Italy) gave an overview of how to extract relevant information from published literature, with a special focus on metadata related to covariates affecting disease emergence. Since data retrieved from literature are often complex and tricky to explore, the practical session showed the participants how to organize them into relational tables in order to build customizable and ready-to-share dashboards, which allow to efficiently visualize and summarize the information collected.
In this tutorial, Mathieu Roche (Cirad, France) Nejat Arinik (INRAE, France) and Mehtab Alam Syed (Cirad, France) first presented an overview of NLP (Natural Language Processing) approaches in order to mine media data for EBS systems. The second part focus on textual classification issues based on data science approaches. Finally, original representations of results are presented for highlighting new knowledge for EBS systems.
ProMED is a longstanding informal disease surveillance network. It has a worldwide network of clinicians, who send in reports of any unusual health events in plants, animals, or humans. These reports are then vetted by subject matter experts at ProMED before being shared with ProMED’s subscribers. ProMED emails usually contain a wealth of quantitative information about outbreak events. However, this information has so far not been utilised in real-time outbreak analysis. Using the West African Ebola epidemic as a case study, Dr Sangeeta Bhatia (Imperial College London, UK) demonstrated the challenges of using data extracted from ProMED for real-time analysis, with the use of a cleaned data set for the same epidemic that was collated by the World Health Organization as a benchmark to understand what can be inferred in real-time using digital disease surveillance data. Data and code are available at https://www.nature.com/articles/s41746-021-00442-3
In this block lead by Tom Hengl and Leandro Parente (OpenGeoHub) participants learn how to use state-of-the-art Machine Learning algorithms in R (mlr, mlr3) for the purpose of building models and producing spatial and spatiotemporal predictions. We used some of the disease datasets and covariate layers (MOOD study area) mentioned in the previous sections, then show step-by-step how to run spatial spatiotemporal overlays, optimize models, run model diagnostics, produce and visualize predictions (as maps or animations). The block is based on the R bookdown: https://opengeohub.github.io/spatial-prediction-eml/