The early detection, assessment and monitoring of a hidden pandemic of antimicrobial-resistant infections
Disease description
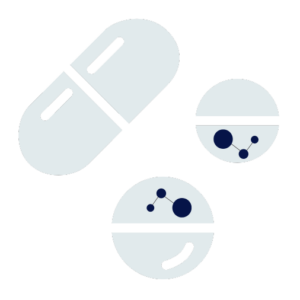
The emergence and spread of drug-resistant pathogens have led to antimicrobial resistance (AMR) now being considered a major public health concern. AMR occurs when bacteria, viruses, fungi and parasites adapt, resulting in these pathogens no longer responding to commonly used drugs. This makes infections caused by resistant pathogens harder to treat, increasing the risk of severe illness and death. Drivers of the emergence and spread of AMR are multi-sectoral, and relate to, among other factors, antibiotic exposure in humans, animals and the environment, standards of infection control, and international travel and trade. To date, AMR surveillance in Europe and elsewhere is mainly relying on indicator-based surveillance. ECDC and national governments launched One Health programs for AMR, involving in addition to monitoring AMR in humans and animals (including food products), environmental surveillance, such as sewage monitoring. Nonetheless, the level of implementation of the One Health approach for AMR between European countries is variable, and the intersectoral evaluation of data originating from these multi-sectoral surveillance remains challenging.
Disease profile
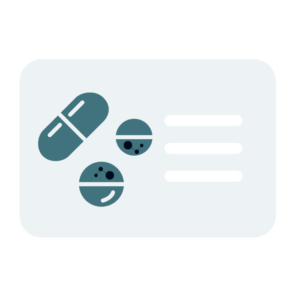
To reach a comprehensive understanding of the factors affecting the ecology, distribution, and trend in Europe of the infectious diseases included in MOOD, information from the available literature and expert input was collected and summarized in “disease profiles”. These documents synthesize the available knowledge on specific diseases, such as AMR (In Press).
AMR Covariates Dashboard
Publications

- Predictive Mapping of Antimicrobial Resistance for Escherichia coli, Salmonella, and Campylobacter in Food-Producing Animals, Europe, 2000–2021, DOI: 10.3201/eid3001.221450
Risk maps
Fig 3: Mapping of predicted P50s and hotspot areas for antimicrobial resistance of Escherichia coli, Salmonella, and Campylobacter, Europe. A) Predicted proportions of antimicrobials with P50 at 10 × 10 km resolution per bacteria. B) Antimicrobial resistance hotspots (light blue) in eastern Europe, Italy, and Spain. Cutoffs: E. coli, 0.43; Salmonella, 0.23; Campylobacter, 0.6 (95% percentile). P50, >50% antimicrobial resistance.
Case study leaders
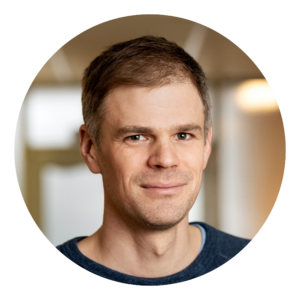
Department of Environmental Systems Science, ETH Zürich, Thomas Van Boeckel (thomas.vanboeckel@env.ethz.ch). Has now moved to the University of Zürich (thomas.vanboeckel@uzh.ch)